Episode 3: Lydia Collett
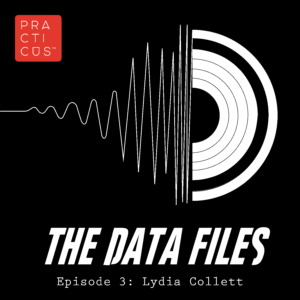
EPISODE 3: Lydia Collett – The Data Files
Lydia Collett (Data & Insight Product Lead at John Lewis Partnership) joins Barry and Tony to discuss the growth of data products. What are they? How do businesses use them? How will product and data combine in the future?
AI Transcription:
Ben Culora 0:02
Hi. This podcast is brought to you by Practicus. Practicus is a recruitment, consulting and advisory business, specializing in change and transformation. We hope you enjoy the podcast.
Barry Panayi 0:12
Hello, welcome to the next episode of The Data Files. I’m Barry.
Tony Cassin-Scott 0:18
And I’m Tony.
Barry Panayi 0:19
And we are super lucky today to be joined by Lydia Collett. Hello, Lydia.
Lydia Collett 0:22
Hello.
Barry Panayi 0:23
Now Lydia’s career has always been in data. But it’s super interesting, from working in one of the oldest industries London market insurance, Lydia moved through Ernst and Young doing data science into the digital native company Zoopla and is now the data and insight product lead at the John Lewis Partnership. Thanks for being with us, Lydia.
Lydia Collett 0:43
My pleasure.
Tony Cassin-Scott 0:44
So Lydia, I was a little baffled when I saw the title of this podcast, what what is a data product?
Lydia Collett 0:51
I guess let’s look back to how the data function evolved. And we’ve been answering questions and service of the business for bloomin long time, right. And that’s how we all started service function. Bob wants to know how many cucumbers he sold in Waitrose. And then Joe wants to know how the sales are doing and the latest John Lewis. And we answer the questions and we answer the questions time and time again in service. But actually, at some point, these questions start to compound. And actually, you need a bit of an ongoing enabling capability to evolve away from just answering questions.
Barry Panayi 1:22
Are you just saying it’s a report? Is a data product or report?
Lydia Collett 1:25
I would say a data product is a product that is ingrained in using data to create reusable capability. So hypothetically, you could call a bit of a report a data product, it’s not a very advanced one. But if your reporting is moving into self serve, then actually you’re helping with decision making. With an always on capability, you could put some buzzwords around it and call it a data product if you tried.
Barry Panayi 1:52
So do you find then that people expect you in your job now to be building AI and machine learning algorithms and calling them data products? Or do you find it’s more the how many cucumbers did we sell type question?
Lydia Collett 2:05
So there’s a variety of use cases in the business and you do not need AI to answer them all. And also, you don’t need to call it AI all the time. Sometimes it’s just like a fancy little linear regression model. So I would say there’s a variety of answers, we do need to help our data science teams in businesses, including ours, get their models out into production, and help users understand what those models are doing, instead of just throwing out predictions to the wild and hoping for the best. So that’s part of it. But then if you don’t have the foundational capability, do you need to sort your reports out. So half and half.
Tony Cassin-Scott 2:41
Is it a decision support tool with some inference built in with expert analysis? Is that that sort of thing? Or?
Lydia Collett 2:48
Yeah, so I think there’s a stage that’s definitely decision support. So if you think about outside of my industry, Google image search. So when you put an image into Google, there’s some clever smarts in the background, right? That go, oh, okay, I can take this and see that it’s a cat. And there’s this and it gives you stuff back. So that’s decision support. But then you can also move into data products that are automated decisions. And doing so for you. It’s basically recommendation systems, right? So when you look at Netflix, recommending movies back to you, and Spotify doing your Discover Weekly, that’s the kind of step on from what we were talking about, but also still data products.
Barry Panayi 3:26
You talked about a couple of pretty interesting data algorithms there. How does it work with your data science teams? I know that you are, amongst other things, a data scientist, you teach big companies how to do data science even. So how does it work? Where you come in as a product person? Yet there’s an established data science function. Is there any sort of, beef?
Lydia Collett 3:49
I, having been a data scientist, but not typical one, have a pretty strong hypothesis that data scientists just want to build machine learning models, like they love to code. They’re technical. They absolutely love it when Google and Microsoft are releasing all these new models, and they can learn about them. So when they’re told when they come into a business, that actually their job is to do a bit of data engineering, and then do a bit of data science, and then create like a dashboard on top of their model. So someone over there can actually use it, they’re like, Please stop, I just want to build data science models. But in business, we need to put that wrapper around them. So we can use them as products and people can understand what they’re saying. So actually, I think it’s the opposite Barry. They want someone to take all this other stuff off their hands. And when we take the stuff off their hands and spend time with the users in the business, who actually making decisions from the models, and we get better relationship with them. It means their work can be usable, and it can be scaled, and they get an even bigger like a thumbs up from the boss that they’re saving more money making more opportunities.
Barry Panayi 4:53
Do you enjoy doing this more than when you were doing pure data science? It’s hard. Okay. For the benefit of those listening is a big smile on Lydia’s face.
Lydia Collett 5:03
So, when I joined consultancy at EY, I went in wanting to be technical and go super technical hands on keys, I love to code. And I didn’t get that but chased the opportunities down and did a stint in Berlin in a software development team. And I was a data scientist working full agile, all I did was data science. And I actually found it really lonely. And I am quite extroverted. For those that know me. I like to talk. I like to speak to people I like to collaborate. And when I was hands on keys, data science, not only did my boss at the time tell me, I wasn’t ever going to be a great data scientist who was super technical. I found it really lonely. So finding that niche between data science, and talking to people and design and creativity. I think I am happier. I think I do prefer it.
Tony Cassin-Scott 5:55
I’ll use the term that sounds horrible, end users, but your colleagues who benefit from from these tools, what reactions have you had from them? Because I suspect for some of them, it’s new. For some of them, it’s, I don’t know why you’re there. And for some of them, it’s going to be like really exciting. And where had you been all my life? What what sort of spectrum? Have you have the reactions been?
Lydia Collett 6:15
I have I had one, where have you been all my life from a guy rolling out ML ops, internally, I quite enjoyed that one. But I think it’s, you’re opening up the world of technicality, data science, analytics, bringing different datasets together to people that are non technical, and you’re bridging that gap. And they may have historically been scared by our field, there’s a lot of people that go oh data, even now they still do it. So when they see the power of what it can do, they’re pretty excited. Or they do go, oh, there’s a lot of work in design here. And oh what about all the maintenance, and you’ve got to take a big step back and invest a lot of time and effort to do this. Can’t we just answer all the questions and stay in the world we are cause I quite like having someone on my side, like answering everything I need.
Tony Cassin-Scott 7:03
That’s an interesting point. Because what you’re doing is it kind of creating a kind of self service world. And I suspect some of those people aren’t used to that self service, they’ll have someone to go to. So all of a sudden, you’ve taken that away, albeit you’ve given them some self service tool, but I’m not sure everyone embraces that do they? Or do they?
Lydia Collett 7:21
No, I don’t think they do. And I think this comes down to another topic outside of products that I’m really passionate about at the minute, which is data fluency. And when people don’t know what on earth, this industry is all about, and they don’t care about datasets, and then don’t get excited when they start to figure out all the possible stuff you can do. They don’t care. They didn’t want to do it. But I think if you can get them to understand. And it sounds really corny, and I don’t want to say it but the power of data, then they buy into it, and they want to be a part of it. And it opens up career paths for people if they do move out of the current. Here’s a report, here’s a number world and they get involved.
Barry Panayi 8:03
That’s it. That’s a really interesting point. Do you think so this whole data fluency, which you carefully didn’t call data literacy, consciously, therefore, implying loads of people are illiterate. Do you think the community upskilling, whatever you want to call it stuff sits nicely with product, as opposed to how it used to maybe sit awkwardly with more technical data scientists? Is that something you’re seeing success with?
Lydia Collett 8:30
Every time we roll out a product, we need to think about the enablement really carefully. We can’t not roll a product without thinking about enablement. So therefore, you do have a sweet spot to roll it in. When you roll out a product, you’ve got a group of users who all care about the same thing, they’re using the same thing, they need to know the same thing. So therefore, you’ve got an opportunity to teach them and you’ve got an opportunity to upskill them in their data fluency at once. Whereas I think, in the places it sat previously, it’s like, we’re going to do data fluency. And you go out to the organization, and you put a big scheme in place, and you kind of hope for the best, but people haven’t necessarily got a reason to use it. You’re just doing it because you know, you need to do it. But if there’s a product that they need to use, it gives them that cause to kind of rally around and a reason to get involved.
Tony Cassin-Scott 9:20
One thing I’ve come across around the data fluency or the lack of it, is storytelling. A lot of people are good at presenting a chart. When I say good, it’s like, here’s a chart, with little interpretation of what’s going on. Where’s the line between the products that you deliver to your customer, internal customer base and the ability to tell the story from the data.
Lydia Collett 9:42
The way I think about this one is actually using an analogy of the weather. So you’ve got this explanatory versus exploratory balance when it comes to storytelling and everything we do in product. So if you think about all the different ways you can consume weather data to know if it’s going to be sunny or rain tomorrow, we love that in the UK. On one end of the spectrum, you can pop BBC News on and Tomasz Schafernaker will explain everything to you. He will tell you the story of the data that is explaining what’s going to happen with the weather. That’s one end, it’s completely like spoon feed, I don’t have to think I just listen. Then in the middle, you’ve got like the BBC Weather app that you can go into. And it explains it a little bit with some charts and graphics, and you need a bit more data fluency. But you can explore it a bit more. And then the other end of the spectrum, which is where I go believe it or not, as a data professional, you’ve got all of these maps and the like rainfall falling over the UK, and you move the sliders and you see what’s going to happen. And they put the predictability of stuff in here. And that is super exploratory down the other end of the spectrum. And because products are built for use cases, and to solve business problems, sometimes we will need to properly tell the story. And we will need to put something like Tomasz Schafernaker on the news presenting the weather and spoon feed, especially if the data literacy isn’t there. But the more you move to exploratory, the more you can start to kind of democratize out the knowledge and the less you need a central hub.
Tony Cassin-Scott 11:08
So the use of the data in itself is a form of data literacy, education.
Lydia Collett 11:12
100%.
Barry Panayi 11:13
I’m very interested in the diverse industries that you’ve worked in. You’ve kept a similar focus data science moving to product and blending it together. But I’m not sure you could have picked different in my perception of Lloyd’s of London insurer is an old guy with a briefcase rifling through paper, making gut feel decisions. Then you moved to Zoopla, which I imagine probably had a slide and a ball pit in the office or something equally gruesome. Are there common themes and challenges that you’ve seen across all of those different industries? Is Question A and in terrible interviewer style, I’ll do a sub question B. What’s the biggest miss that you’ve seen?
Lydia Collett 11:57
The common themes, and it might be because of the time and like, what’s going on in industry at the moment is excitement around data science, and the buzz wording of everything. I’ve been in the prime moment of everyone going big data. And everyone going AI, especially in consultancy, I love consultancy, but my god did we overuse AI. So I think the buzz and the excitement, I’ve definitely seen everywhere, whether they knew what to do with it or not, is a different story. And then something I don’t think people have got quite right is the balance between experimentation and actually getting stuff into production, that’s adding business value. So when I look at insurance, and Lloyd’s of London old industry, we did a lot of cool experiments, we were doing actually seem like pretty good, high caliber stuff. But none of it got into production. Because every time we went out to the business, they were like, well, they didn’t say this, but this is what they’re thinking. I’m retiring in five years time, if you implement this, I’m not going to have a job. And I don’t understand what this geospatial analytics thing is. And actually, my gut feels pretty good. Because I’ve been doing this for 10 years. So your experiments never got off the ground and into prod, no matter the people you had. When I think about consulting, they, I mean, outside of consulting, when I was an internal product development there. I was working in internal AI product development. They again did loads of cool experiments, and they built stuff properly got it into production, but they didn’t get the business casing right. Zoopla other end of the spectrum completely. So this is why no one’s got it right so far in my book. So they did all their experiments, didn’t get a single data science model into prod. And then when something’s not quite right here, and rebalanced. I think, and I’m saying it cos it’s my current role, but I think we can get the balance a bit better where we are. We’re investing in ML ops to get our models into prod and data science. We’re building a data product functionality, we’re taking a step back and going right actually, we’d need to stop being a service function and like, think about how we identify opportunities ourselves and proactively deliver. But if we don’t get that business casing right and identify the right opportunities upfront, we’ll build a whole load of cool stuff, and no one will use it and no one will see the value and then I’ll be out of a job.
Tony Cassin-Scott 14:22
But it sounds like your purpose is is embedding, embedding skills capabilities through insight and what have you. So are we going from a world where something is new, where something will be embedded as BAU? And if that’s true, what does the data function look like in the future?
Lydia Collett 14:40
I think, yes, we’ve been new in the past and people didn’t know that you could create a Tableau or Power BI dashboard. And then they realize actually, it’s quite easy and you don’t need to have 20 years of experience to do it. You can learn it in a few weeks or months. So that gets embedded but then I think, as the stuff that was new before is embedded, there’s always going to be new bits coming through. And organizations are all on different journeys and levels of maturity, which means actually, the data science stuff that’s becoming BAU for some companies is becoming new for others. And then once they go beyond that, maybe they’ll go into deep learning. Like there’s always something new in the industry, there’s always like those frontline researchers who are doing new stuff. And that will always be at some points new for us to embed. But at what point does the data function stop? And that’s technology beyond just data? I haven’t answered that one yet, in my head.
Barry Panayi 15:34
Plenty of time, I’m quite keen that the whole industry doesn’t implode quite yet.
Tony Cassin-Scott 15:39
Well, for all our sakes. So I mean, just picking the theme and the role of the CDO (Chief Data Officer), I mean, I’ve come across industries where they’ve kind of bundled data in with other experts skills, such as product development, or IT or both. Do you have a view on that? Have you seen that? Does it work? Is it too early?
Lydia Collett 16:00
Again, it depends on the maturity of the organization. I think if you’re hopefully not right now, this is the case. But some organizations are still only just realizing that you need to pay attention to your data, then you need someone to get data on the agenda. And if you embed that in another role, you’re kind of running before you can walk, right? You need someone to shout from the rooftops like, folks, let’s talk about data, like it’s important. It needs to be at C suite level. And you need to get your pipeline of talent coming in, you need the new grads in the industry to understand that you’re taking this seriously to recruit. But once you get beyond that point, and your C suite and more data fluent, and they understand how data is being used. And you’re moving towards creating products rather than answering how many cucumber questions, then I think there might be opportunity to merge. And I haven’t seen it anywhere yet. But Zoopla, we as a data team did report into the chief product officer. So we had a product and tech function. So all of the development for the app and the website, sat alongside us as a data team. We had analysts embedded in product teams developing for the app and the website. And we worked as one or reporting into Chief Product Officer, no chief data officer. And because he got data, and because he spoke for us, and we had a great director of data and analytics, who could also speak with him. It worked but digital native, they don’t have all the legacy to deal with a little bit legacy, but not anywhere near as much as 150 year old retailer, they could get away with it. And it was working.
Barry Panayi 17:36
So going on to that 150 year old retailer, which we both know very well, what do you think the biggest opportunity for data and insight products is in retail? Because the reason I joined retail is because it’s so fast moving, and there’s a lot of data and you can do something and results change quickly. Why do you do product in retail? Why did you choose you could have gone anywhere? Or why did you choose to go into retail? Don’t say because of the staff discount? Although it is pretty good.
Lydia Collett 18:04
Well, I don’t want to say because I like shopping because I don’t want to sell that part of me. But a little bit of the answer is actually that. So I think retail is one of the simpler industries, we buy things, we make some things, we grow some things and we sell them. And I can comprehend that business model. And I can see the opportunities in it, having not worked in it for years. Now if I compare my time and consulting, why did infrastructure, energy, I didn’t do much oil and gas, but I veered away from it. I didn’t, I just couldn’t comprehend how you get oil out the ground and have to deal with that. There’s some cool opportunities there. But I think you’ve got this piece about enabling people who work in retail and may not have a data background to use it. And you’re getting out there and you’re helping them and helping them upskill I love that side of it. But also that all the supply chain and the operational side of things, it kind of fascinates me. Like we can all relate to retail, we all buy things, we all go to the supermarket. And that means that you can relate to the journey of how did that tin of baked beans get onto the shelf into your basket, and then you cook it for dinner for your kids. And helping people optimize that. I remember my optimization module at uni and I absolutely loved it. There’s a little bit of a kind of technical geek in me that likes that side of things too.
Tony Cassin-Scott 19:24
The reality of it all.
Lydia Collett 19:25
Yeah, you can see the impact, can’t you. You see the trucks arrive more quickly. You see the cages filled optimally versus like half filled. You can then go into the branches and the shops and actually speak to the people doing it and see how you’ve improved their lives and made them happier. So yeah, I think it’s the relatability of it, and it’s ripe for it. You can talk about all of the like having augmented reality installs and all of that jazzy stuff and yeah, we’ll get there but actually, there’s some really cool operational stuff that’s quite fun to explore.
Barry Panayi 19:58
Very good. You’ll be pleased to know you’re almost at the end of your time in what we’ve called the data dungeon down here. Again, you won’t be able to see on the podcast but dungeon like as it’s done, it’s certainly dungeon like, yeah, and freezing cold. I think there’s a 20 degree temperature swing between here and outside, we always ask our guests the same question. So here we go. What is the best advice you never took?
Lydia Collett 20:23
When I was in education, and even now I’ve always chased success and achievement. And there was a bit of me when I was at college and uni, I was like, I need to be successful, I want to be that business person. So I chased it. And I was like, investment banking is the one for me, I went down the route, I did all of the internships. And I ultimately didn’t go into it coz I didn’t get an offer at the end of my internship. So like, there was that. But my mom said to me, like, use your creativity and go after something that you really love. It feels like you’re working a lot for the status and you come home and you’re exhausted on the weekends after doing PowerPoint at 2am. Genuinely, the magic roundabouts when you left and came straight back in a taxi, and just got changed in her shower at home. And you don’t know any different when you’re a grad. So she told me to chase the creativity. And it’s taken me a little bit of a few years and a few different jobs to start to realize that actually, that’s okay. And I’m starting to get into it now and exercising that at work and then also finding hobbies that I can do outside of work. So yeah, I don’t need a linear career path. I didn’t quite get that straightaway. And my mum knew. She was a head teacher of a nursery school, but she knew that that was the case.
Barry Panayi 21:39
That’s really interesting. There’s this concept, the squiggly career. I don’t know if you’ve seen though, Helen Tupper. I’ll give her a different credit. You can buy me a cup of tea, Helen. And that’s a real thing. And you describe that really, really well. And in terms of success, of course, the pinnacle of everyone’s career is appearing on The Data Files podcast. So check that one off. Thank you very much, Lydia. That was amazing. We really do appreciate it.
Lydia Collett 22:05
Thanks very much. Pleasure to be here.
Tony Cassin-Scott 22:07
Thank you for coming.
Transcribed by AI