Episode 2: Rachel Purchase
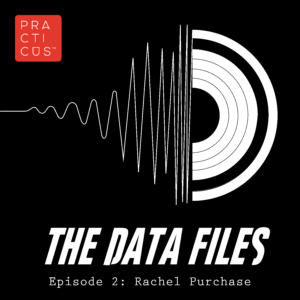
EPISODE 2: Rachel Purchase – The Data Files
Barry and Tony are joined by Rachel Purchase (Head of Data Product, Insights and Data Science at Admiral). We discuss some of the ‘myths & legends’ that have surrounded the data profession and what the reality is like for most businesses.
Use our highly-rated data resourcing services to build your data leadership capability and reach your business ambitions.
AI Transcription:
[00:00:00] Ben Culora: Hi. This podcast is brought to you by Practicus. Practicus is a recruitment, consulting and advisory business, specializing in change and transformation. We hope you enjoy the podcast.
[00:00:13] Barry Panayi: Hello, Welcome to the Data Files. I’m Barry.
[00:00:16] Tony Cassin-Scott: And I’m Tony.
This is the second our series on the data Files Myths and Legends. Or they’ll like to think about it difference between facts and fiction.
[00:00:25] Barry Panayi: We’re very lucky. To have with us a special guest, from Admiral, Rachel. Hello. Hello. You’ve got one of the most impressive titles of the people that we’ve seen here on the podcast so far. Tony, can you read it out cuz I have my pen ran out.
[00:00:39] Tony Cassin-Scott: I, I, I’m, I’ve had to get a wider screen, but it’s Head of Data, Products, Insights and Data Science. Is there anything that’s missing?
[00:00:47] Rachel Purchase: It is about the longest title known to man. It means that I cover most of the data world within Admiral, but not things like data governance and data assurance.
[00:00:56] Barry Panayi: So Rachel, there’s a lot of talk in data teams around what the biggest challenges are, and one thing that keeps coming up is culture or the data job is more about culture change.
It’s more about understanding the right questions to ask and all of the soft and important elements like. Do you buy that as one of the biggest challenges or if I can be so bold? Is it an excuse that we as data people tell each other because we haven’t quite landed our strategy? Where are you on that?
[00:01:29] Rachel Purchase: I think probably both.
And so the first kind of analogy when you ask that question that comes to mind is airline flights and you, you go, Who’s been on a plane recently who’s had a good experience, put your hand up and everybody complains that they haven’t had a good experience. If you’d asked that question 50 years ago, you’d have a very different answer cuz everybody would’ve had a wonderful experience because it was such a novelty to, to do those things.
And I think data is suffering from the same, sort of impact right now. You know, the expectations are growing faster than the teams can keep up. So in most companies, I don’t think they’ve failed, but I think they probably have unrealistic expectations about what they should be able to do right now. Because actually if you go back five or 10 years, they probably made huge inroads in terms of their sophistication with both data, analytics and all of that stuff. But as soon as you know, somebody sees a little bit of it, it’s like, Oh, we want that everywhere now. We want more of it. And you normally can’t keep up with the demand.
Of course there are ways of improving that. Culture is a big part of it. If you get that kind of culture and the ways of working, right, you do accelerate that journey. And I think that’s well known now that, you know, you start with a business problem. You embed the business people, you make sure you take them on the journey with you and work very closely together. And I think sometimes it is still failing in that you’ve still got these siloed teams and, and to your point, you know, data are using culture as the excuse, but really what they’re doing is probably not enough with the stakeholders, not enough with the business and not enough about that education piece.
Maybe I see it slightly differently and that I don’t see data as failing, but I do think that expectations are probably out stripping the reality of, of what can be achieved.
[00:03:07] Barry Panayi: Have we hyped this up too much too early? Have we been too successful at selling the benefits of data five, 10 years ago? And now we’ve done some low hanging through delivered value early. We’re getting to the crunchy bit. Yeah, I’ve interpreted that. Right.
[00:03:22] Rachel Purchase: E, exactly that. And I think this happens in a lot of places. You go from that first use case where you go, Look, let me show you what machine learning can do, or Let me show you what I can do here. And then suddenly, once you’ve made that a success, you’ve got 10 use cases coming behind it, but perhaps you haven’t necessarily.
Productionized your first use case yet you haven’t really got to that point where this is a well oiled machine and there’s a lot of that sort of foundational work that needs to happen to really make that work for an enterprise.
[00:03:48] Barry Panayi: So there’s something on maturity there around, you had a little look behind the curtain. People have seen that first value delivered that we’ve lashed something together that’s worked, but there’s almost a stepping back and hardening things that perhaps is something that people aren’t appreciative of. Because I guess the myths and legends theme, the myth would be you hire these people, they’re excellent and they’re gonna deliver loads of brilliant things. And then in day one, week one, year one, you’re delivering amazing things and then suddenly it’s incumbent on the leader. To put the brakes on to make sure we build the right foundations to almost productionize all of that.
And perhaps that’s what, that’s a message of this podcast I guess, that your brilliant answer has just told us, which is we show a chin of light of what can be achieved, but not everything is that easy if you want to do it, uh, industrialized.
[00:04:43] Tony Cassin-Scott: I think there’s a issue of being a victim of success here cuz you’re able to show the art of the possible, with few resources because you’ve just like razor sharp and you’re focused on it. But to industrialize that there’s a requirement for investment, there’s a requirement for some time for r and d for to expand that, that proof of concept, is that understood or is it just say, Well, you just did this in like a day. Why can’t you do the next one in a day?
[00:05:09] Rachel Purchase: Yeah. I think some people understand it better than others, but it’s definitely an education piece and a that you have to go on because people don’t really. Think or care, and they shouldn’t have to about all of those foundational areas. You know, they don’t necessarily see the value in things like the data catalog and making sure that we’ve got really well governed data that we can deal with, you know, PII and all of that stuff. But ultimately it is fundamental to enabling that end value and it, it’s a classic thing, isn’t it? That everybody says you, you put rubbish in, you get rubbish out. But really all your kind of end business user is interested in is how do I move the dial on this metric? How do I make my business go faster? So you’ve really got to. Try and bridge that gap with them without getting into too many technical details, but explain, this is the reality of where we are. This is where we want to take you, but this is what we have to do. And it probably requires more investment than they’re expecting.
[00:06:05] Tony Cassin-Scott: I mean, what I find interesting about that is that the, if you, for, for financial reporting, for example, which is a regulatory requirement, people understand the requirement or the quality of the data in order to meet those needs. But you know, when it’s non-regulatory, when it’s a, a business product that you’re providing, all of a sudden that goes out the window. Why is that?
[00:06:24] Rachel Purchase: It’s one of those weird things, isn’t it? Because they absolutely do, because they know the importance of regulatory. They know they’ve gotta get it right if they’re submitting that information. But yet, when it suddenly comes to, Oh, what can I do over here with business decisions? It feels somewhat different. And I think some of it is that data is in that weird place, isn’t it? Where it’s quite easy to do in some ways, but it’s really hard to master. And so you have quite a lot of people across the organization who think they know how to do it. Again, it’s that education piece. You end up with people going, Well, I can build this over here in SaaS in like a day, but that’s a completely different thing to having a fully engineered product that is supported, that’s meeting the 99.9% SLA and so on and so forth. So I think when it comes to the business side of it, you have people who are excited and they’re energised and that’s great. And they really want to get the value out of data. And they can get their hands on things that make it seem easy. Uh, and you’ve gotta find that balance between where do you need that rigor? How much of it do you need? And trying to explain to them why engineering is different to doing it over here in a sandbox.
[00:07:31] Tony Cassin-Scott: I mean, is that a cultural piece, do you think? Because one, you go to jail metaphorically anyway, if you fail to supply the data to hmrc, whereas the other one, you kind of get some business damage, but no one goes to jail. But that, I would say they’re equally important.
[00:07:48] Rachel Purchase: Yeah. Although I think that what will happen is that people think they will get a business advantage by being able to do it quickly themselves and so on, so they don’t see it as, I’m going to hurt the business. They see it as actually I’m agile. I can be really quick. I can deliver something that has value, and sometimes that is okay. I mean, it’s not to say that everything has to be perfectly engineered, but I don’t think they see it as go to jail, hurt the business. I think it’s go to jail or I, my option is do it quickly or wait over here for something that’s gonna take a long time. And they see that long time almost as hurting the business because there’s the opportunity cost of being slow.
[00:08:24] Tony Cassin-Scott: I guess it’s horses courses and if you’re producing an automated vehicle, you probably wanna get that right and not, not try that iteratively
[00:08:32] Rachel Purchase: Indeed. Although, you know, you can do everything iteratively, but we won’t get into a discussion on that job. But you know, they can, bits of it can be automated first.
[00:08:39] Tony Cassin-Scott: It’d be interesting to get your views if you’ve seen any companies that have adopted the use of data alongside changing the tech and the culture as part of a sort of a big program or change. And whether that’s been successful or not.
[00:08:51] Rachel Purchase: There’s one that stands out when you ask that question, and I’m not close to it. I’ve only seen sort of, you know, the, the advertised material and so on, but is the financial times who’ve gone from being kind of, you know, a printed newspaper to being completely online. And actually now they offer kind of data services to other publishers to, to help them understand how to do it, where the best practice is. And you know, they talk about some amazing kind of results that they’ve had by going on that data journey and, and really sort of, digitizing their business and really investing in data. And, you know, they’ve gone from probably like zero online presence to, you know, I think they say now they have like over a million paid subscriptions on on their website. So I think they’ve free. A, a great job in that space because it’s not your sort of typical arena for it either. I mean, I guess 10 years ago when they started, you wouldn’t have been thinking hate newspapers really need to invest in digital and data and, and tech.
[00:09:50] Barry Panayi: It links into that. You talked about the data being sold as a service by the FT and all these are products as well as revenue streams. And going back to your job title, it had data product in there. And I have a data product team too, but I guess it’s quite a new term. So I just wanted to check and get your view on what is a data product in your world.
[00:10:12] Rachel Purchase: This is a great question and. It’s something that, you’re right, it’s quite new and it’s, it can be sometimes quite hard to describe to people.
So just for, for clarity in, in my world, everything that we do is internal. So we don’t sell our data externally or anything like that. So all of our customers really are, are internal business customers. But what data product means from our perspective is very much that you are treating data like it is a commercial product. So you know, you have a product catalog, you have a product owner who is responsible for maximizing the value of that product. So, you know, they should constantly be looking for ways of getting that data into the business. Are we maximizing the use of it? Do all the right teams have access to it? All of that stuff where they’re treating it e exactly like you would a product that you’re going to sell on the shelf, except that they’re selling it to the business. And then they’re also obviously working with the development teams to go, Okay, well this is what we need next. This is what we need you to do. This is how we’re gonna improve the value that we’re driving from this data. And probably the bit I should have said first and and missed in the explanation is that you have sort of two different types of data products. So you have the data itself, and that could be, you know, the raw data. It could be your sort of derived data, your algorithms or insights or whatever it is that you’ve come up with. And then you’ve got the delivery mechanisms for that data. And we put all of that under data product. So those delivery mechanisms could be an API integration, it could be a dashboard or whatever it is. And you have to have product owners essentially owning. Those products to maximize the value across the business and making sure that you have reusability and that they’re discoverable and all of that good stuff.
[00:11:56] Barry Panayi: So these, um, data product owners, would you say they’re data people who have been trained, cross skilled to become product owners? Are they died in the wool product owners who’ve lived and breathed agile that are learning a bit about data or wherever you found them?
[00:12:15] Rachel Purchase: Both. And actually that works really well in the team. So when we started, we very much started with data. People who knew not just data, but knew admiral’s data. So they knew the domain, they knew the business, they knew all the people. So they had a lot of that stuff set up ready to go for them. In terms of, you know, being able to manage the stakeholders and all of that. But they didn’t really know Agile and we had to do a lot of training around Agile, how to break down stories, the vertical slicing, all of that stuff. And then over time we’ve also added in people who we’ve hired in who may have great product knowledge that, you know, real product experts. But they haven’t necessarily been that close to data in their previous jobs.
And actually that balance works really well because as a team, it means that they can help each other with both aspects of the job. And we get better in, in both domains, not just kind of knowing the business and knowing data, but also in being real agile experts and knowing all of that side too.
[00:13:12] Barry Panayi: How’s the rest of the business admiral, outside of your directorate reacted to you having product? Is there ever any confusion with someone in motor saying, When I own the my motor product or I own this product?
[00:13:26] Rachel Purchase: Lots of confusion and it’s actually something that we’re probably suffering from more right now than, than maybe a few years ago because, What we did a few years ago is we decided to do this transformation program, which we called dna, so data and analytics, and we sort of ring fenced that and that’s how we stood up data product was in this sort of, I don’t how you describe it, but this nice sort of you, we had not, not the walls up because we were inviting the business in, but we had a very clear mandate. We were left alone. We had the autonomy to kind of just start from scratch and say, Hey, let’s build a brand new data platform. We didn’t do a migration. Started again on, on gcp, and now we’re sort of at that point on that journey where we’re going, Well actually it’s now gotta fit in with everything else in terms of the value streams and how we structure the whole business as agile. It can’t just be the standalone so program anymore. We’ve gone past that point. And that’s where these conversations are now coming in and going, Well, hang on. I’ve got a product owner over here for this value stream. Why would I need your data product owner as well? And, and all of that stuff is essentially what we’re working through right now.
[00:14:31] Barry Panayi: I’ve heard that elsewhere as well. Sorry, Tony. No, no. I’m not that sorry.
[00:14:35] Tony Cassin-Scott: You never are. We be sure you mentioned Tony about expectation management. About what’s possible and what’s hard and what isn’t possible. I’m thinking about the role of the product managers that you’ve got now and the way that they educate the non data people within Amal, whether they have a role of managing those expectations about what is reality and what isn’t.
[00:14:57] Rachel Purchase: Yeah, they d they definitely do. There are also other people who have that role too. I mean, yeah, we do it a lot from the data science teams. We do it a lot from the MI teams, but they, they definitely. Add to that conversation, and they make sure that they’re managing those expectations in terms of the reality of what we can deliver and when and how fast it’s gonna go. But also, you know, maybe how accurate that model might end up being, because that’s another. Big thing, you know, And there’s so much hype out there, so many people trying to sell you stuff that they say this is gonna be, you know, 99% accurate. And everybody thinks that means it’s gonna solve every problem they’ve got. And trying to, to manage all of those expectations, certainly products play a part in that, but they’re not the only ones. I guess in, in that message.
[00:15:40] Tony Cassin-Scott: Something which you may or may not have thought about, but how much time do you actually spend managing expectations about, Well, you just example there, you know, this is gonna fix all my problems, but actually it’s not. So how much of your time would you say is actually spent telling people actually know the reality is this?
[00:15:57] Rachel Purchase: Actually not as much as maybe you might think, but I think that’s because of the business that I work in. So Admiral is ver like always being a data driven business. You know, I joined 13 years ago and even at that point, you know, you had, um, people like David Stevens, who is the COO at that point, would be very hands on with data, very data literate, and that’s part of what’s always made us successful is that all the way through the stack, senior management have been very, very brought in to data. And so you still have to do it. It’s not that to say that, you know, we’re perfect and we don’t have these challenges, but perhaps not so much as maybe in other organizations where they haven’t. Grown up knowing that data is fundamental and you we’re an insurance business, so it always has been. We wouldn’t have been able to price a policy or, or manage a claim without it.
[00:16:44] Tony Cassin-Scott: Looking at your background, I mean, you’ve sort of educated statistics and maths and what have you, so it’s, it’s kind of, you cut you in half and I’m sure it says SPSS across you or something like that. Show my age here. But in your opinion, do you think you need that sort of digital dirt under your finger?
[00:16:58] Rachel Purchase: I think it definitely helps because data is complicated. It is hard, and trying to understand where people are coming from, I think it’s always easier if you speak the same language. That being said, There’s huge parts of my role where I have to understand things that I haven’t got my hands dirty with. You know, especially in today’s world where actually data is becoming more and more like software engineering. You know, DevOps is hugely important. ML op is hugely important, and I, I’m sitting in meetings, you know, with people talking about, you know, the virtue of using Jenkins, or I’m going, I don’t know what this is all about. And so even in. You know, even if you have got your hands dirty in some part of data, you wouldn’t have got your hands dirty in all of it because it’s so broad. So I think it’s helpful. I think it helps build your knowledge base. It helps you to understand the challenges when you’re leading, but I don’t think it’s necessarily a prerequisite that you have to have done that as long as you’re willing to, to learn and educate yourself as you go along. I spend most of my life in when these meetings happen on Google going, does this mean okay. That’s what that is. Yeah. Okay. Kind of linking the threads together, just about enough to follow the conversation.
[00:18:13] Tony Cassin-Scott: We know your secret now.
[00:18:14] Rachel Purchase: How I’m doing the podcast without Google, I mean that, that’s a miracle.
[00:18:17] Tony Cassin-Scott: Yes, put that computer down.
[00:18:18] Barry Panayi: In the land of the blind. Don’t worry, , on that point, I think it’s really interesting. It’s, It is. An angle that we’ve had on this podcast before, because it’s pretty much divided people, and I think it’s a, It is a pretty nice, well balanced answer in that you can have the dirt under your fingernails, as you put it, Tony, but there’s just not enough fingernails because now you know, if you’re doing ML ops and you’re on the more data engineering side and you’ve grown up that way, that’s great, but then maybe you won’t know the science and the analytics, but if you’ve come up through the analytics and the vis and coding credit risk models or whatever, it is very broad, isn’t it? And I think that going back to our myth busting, nothing’s as simple as it seems. You say data leaders, well they’ve done the data job that they know it or they don’t know it. But actually knowing some of it’s quite good.
So if you were giving advice to the chairperson or CEO when they’re hiring a CDO (Chief Data Officer) and someone purports to have done all the data jobs. It’s probably a lie.
[00:19:14] Tony Cassin-Scott: I think there’s a good chance.
[00:19:15] Rachel Purchase: Very good chance.
[00:19:16] Barry Panayi: We’ve got a bit of a off the wall question that we ask everyone that comes down into this data dungeon. What’s the best bit of advice you have never taken?
[00:19:27] Rachel Purchase: Oh, it’s a really hard question that isn’t it? I think it’s probably to have gone and done something different. So Admiral is really big on people moving around the organization, people getting different experiences outside of their kind of, their world.
And I’ve never done that. And I think, you know, a lot of our senior managers and a lot of people across the business have worn very different hats throughout their career. So it looks very different to my kind of straight through data, and you learn so much as a result of doing that. I’ve going outside of your comfort zone and going, Hey, I know I’ve worked in data, but actually I’m gonna go and manage an operational department, or I’m gonna go and do this completely transforms sort of your way of thinking about certain problems and your understanding of how the business works and, I think I’ve known that that’s a piece of advice that’s generally given within Admiral, but, and it’s never felt like there’s quite been that right time to do it and to step out and, and I wonder whether that is probably the best piece of advice that I should have taken, but didn’t.
[00:20:25] Barry Panayi: Still time isn’t there. It leads me like, it’s usually the last question, but I, I’m, Can I throw a bonus in? You are in charge of data for Admiral. Lots of bits of the data, not the governance bits we talked about before. I’m, I’m trying to, You haven’t taken the advice so far. What is the lateral move that would make sense to data professionals if they wanted to broaden?
And there’s a question behind the question , which is, how could the head data person become the CEO of a company? Eventually you’d, you’d think you’d, you’d need to travel a bit around the organization naturally. , Um, what is it? How would we take that advice collectively as data professionals and those data curious on this podcast?
[00:21:08] Rachel Purchase: I dunno, I guess it depends where you are in your career as to what that natural step might be. And there are things that have, I guess, a greater affiliation with data wealth, and so making that move sort of a little bit easier. So things like maybe, marketing, you know, if you moved over into a role like that, a lot of the skills that you’d built up within data are very, very relevant to, to what you might do. Although obviously there’s a lot of skills that perhaps you don’t have either when you, when you’re moving into that space.
[00:21:37] Tony Cassin-Scott: It’s a good point, a good question, Barry, but does it depend on the organization which you operate? So if it’s a technically focused organization, you may get more points. If you got a technology background, or similarly, if you had an insurance background, would you gain more points if you had an underwriting background?
[00:21:54] Barry Panayi: That’s a very good point, isn’t it? I look at one of my previous employers, Amlin, and when. Charles left the chief underwriter, became the CEO for a time enough. Is that kind of the gist, your question?
[00:22:07] Tony Cassin-Scott: Yeah, it is. Is it, Is it specific to the industry rather than the general question?
[00:22:11] Barry Panayi: I haven’t thought it through like that. So what do you think, Rachel?
[00:22:16] Rachel Purchase: I think it must be, isn’t it? It’s got to be in, you know, in relation to what it is you’re trying to achieve in terms of the industry you’re in. And, and just as you were speaking, I was also thinking that as well as, you know, moving in terms of your career, I think there’s also a thought about, is there training, So, you know, external qualifications, MBAs, that type of vein, which actually help you become more rounded as well. So you can talk about. Do you take a, a job and a sideways move in order to then go up with a broader range of experience? But I guess there are other ways of doing it, and, and MBAs are a good one in terms of giving you more of that kind of general business knowledge, if your ambition was to become something like the ceo.
[00:22:56] Tony Cassin-Scott: I as, as an example, I mean, I used to work at the ft so thank you for mentioning it earlier. And I’d like to take some of the credit for that.
[00:23:03] Rachel Purchase: You can have all of it.
[00:23:04] Tony Cassin-Scott: Well, not all of it. That’s unfair. But I will take the, the, the, the initial part of it, the, I mean, the current CEO has been there many years, but his background’s in journalism for a journalistic type organization isn’t unreasonable. But I was there for a few years and he wasn’t the only journalist to become a ceo. There were people who weren’t journalists and backgrounds, but they all brought something different to the party. None of them were bad. But they brought different views and they moved the organization along differently according to their own background. I’m just wondering about how much of this is a point in. And maturity of both the organization and the market. So it’s the right person at the right time.
[00:23:40] Rachel Purchase: And, and maybe if you look forward as things like tech and data become increasingly important, then perhaps the right time will come for, for companies to step somebody with that type of background into that role.
[00:23:53] Barry Panayi: Excellent. Thank you. An optimistic note for all our data listeners out there, there at the end, I think. Yeah. Thank you very much Rachel.
[00:24:00] Rachel Purchase: No problem at all. Thanks for having me.